【佳学基因检测】揭密:基因检测结果与临床表征关系研究的设计
做个基因检测需要多少钱介绍
综述肿瘤基因学知识要点记录《J Natl Cancer Inst Monogr》在. 1999;(26):17-23.发表了一篇题目为《基因表征研究的设计:概述》肿瘤靶向药物治疗基因检测临床研究文章。该研究由D C Thomas等完成。推广了基因检测位点与临床表征关系的研究,进一步强调了基因检测位点选择与验证的方法学的重要性。
肿瘤靶向药物及精准治疗临床研究内容关键词:
失巢凋亡;胶质母细胞瘤,免疫疗法,泛癌分析,干性指数,肿瘤微环境,位点研究,准确性验证,方法学
肿瘤靶向治疗基因检测临床应用结果
基因检测结果中的基因型与表征之间的关系研究小组的这一系列论文解决了旨在评估克隆基因群体特征的研究中的设计问题,例如它们的等位基因频率、外显率、这些参数在亚群中的变化,以及基因-环境和基因-基因相互作用。《基因型是如何决定表型以及怎样才能知道》的文章概述了已提出的各种设计,包括使用独立和相关个体的队列和病例对照设计以及最佳多阶段抽样和混合设计。各种统计(偏差和效率)和实际考虑因素被建议用于评估替代设计,基因解码基因检测的研究目的是提出问题,“什么是特定情况的最佳设计”?这个问题的答案显然取决于诸如基因解码基因检测的研究结果变量的性质、基因频率和遗传相对风险以及基因-环境和基因-基因相互作用的重要性等基因解码基因检测的研究介绍问题。进一步的基因解码基因检测的研究方法学工作可能有助于评估总体上人口分层问题的严重性以及处理它的基因解码基因检测的研究方法,高风险家庭登记的效用,以及各种混合设计在基因发现和基因方面的优点表征。基因解码基因检测的研究方法:使用非负矩阵分解算法对集成数据集进行有效降维。分析了两个集群之间的肿瘤微环境 (TME)、干性指数和临床特征的差异。利用差异分析、加权基因共表达网络分析(WGCNA)、单变量Cox回归、最小绝对收缩和选择算子回归筛选预后相关基因并构建风险评分模型。进行免疫组织化学以评估临床标本中代表性基因的表达。在GBM和泛癌中评估风险评分与TME、干性、临床特征和免疫治疗反应之间的关系。基因解码基因检测的研究结果:根据失巢凋亡相关基因表达确定了两个明确的聚类。分配到 C1 的 GBM 患者的特征是总生存期缩短、抑制性免疫浸润水平较高和干性指数较低。基因解码基因检测进一步构建了一个风险评分模型来量化失巢凋亡相关基因的调控模式。较高风险评分组的特点是预后不良、抑制性免疫细胞浸润和分化表型,而较低风险评分组表现出相反的效果。此外,较低风险评分组的患者表现出更高频率的异柠檬酸脱氢酶 (IDH) 突变和对免疫治疗更敏感的反应。进行了药物敏感性分析,揭示高风险组可能从靶向 PI3K/mTOR 信号通路的药物中获益更多。基因解码基因检测的研究结论:基因解码基因检测揭示了失巢凋亡相关基因与临床特征、TME、干性、IDH 突变和免疫治疗之间的潜在关系,以及阐明了它们的治疗价值。关键词:失巢凋亡;胶质母细胞瘤;免疫疗法;泛癌分析;干性指数;肿瘤微环境。
肿瘤发生与复发转移国际数据库描述:
This collection of papers from the Gene Characterization Panel addresses design issues in studies aimed at assessing the population characteristics of cloned genes, such as their allele frequencies, penetrance, variation in these parameters across subpopulations, and gene-environment and gene-gene interactions. This paper provides an overview of the various designs that have been suggested, including cohort and case-control designs using independent and related individuals as well as optimal multistage sampling and hybrid designs. Various statistical (bias and efficiency) and practical considerations are suggested for evaluation of the alternative designs, with the aim of posing the question, "What is the optimal design for a particular situation"? The answer to this question clearly depends on such contextual issues as nature of the outcome variable, the gene frequency and genetic relative risk, and the importance of gene-environment and gene-gene interactions. Further methodologic work might be usefully directed toward assessment of the seriousness of the population stratification problem in general as well as methods of dealing with it, the utility of registries of high-risk families, and the merits of various hybrid designs for gene discovery and gene characterization.Methods: The non-negative matrix factorization algorithm was used for effective dimension reduction for integrated datasets. Differences in the tumor microenvironment (TME), stemness indices, and clinical characteristics between the two clusters were analyzed. Difference analysis, weighted gene coexpression network analysis (WGCNA), univariate Cox regression, and least absolute shrinkage and selection operator regression were leveraged to screen prognosis-related genes and construct a risk score model. Immunohistochemistry was performed to evaluate the expression of representative genes in clinical specimens. The relationship between the risk score and the TME, stemness, clinical traits, and immunotherapy response was assessed in GBM and pancancer.Results: Two definite clusters were identified on the basis of anoikis-related gene expression. Patients with GBM assigned to C1 were characterized by shortened overall survival, higher suppressive immune infiltration levels, and lower stemness indices. We further constructed a risk scoring model to quantify the regulatory patterns of anoikis-related genes. The higher risk score group was characterized by a poor prognosis, the infiltration of suppressive immune cells and a differentiated phenotype, whereas the lower risk score group exhibited the opposite effects. In addition, patients in the lower risk score group exhibited a higher frequency of isocitrate dehydrogenase (IDH) mutations and a more sensitive response to immunotherapy. Drug sensitivity analysis was performed, revealing that the higher risk group may benefit more from drugs targeting the PI3K/mTOR signaling pathway.Conclusion: We revealed potential relationships between anoikis-related genes and clinical features, TME, stemness, IDH mutation, and immunotherapy and elucidated their therapeutic value.Keywords: anoikis; glioblastoma; immunotherapy; pan-cancer analysis; stemness index; tumor microenvironment.
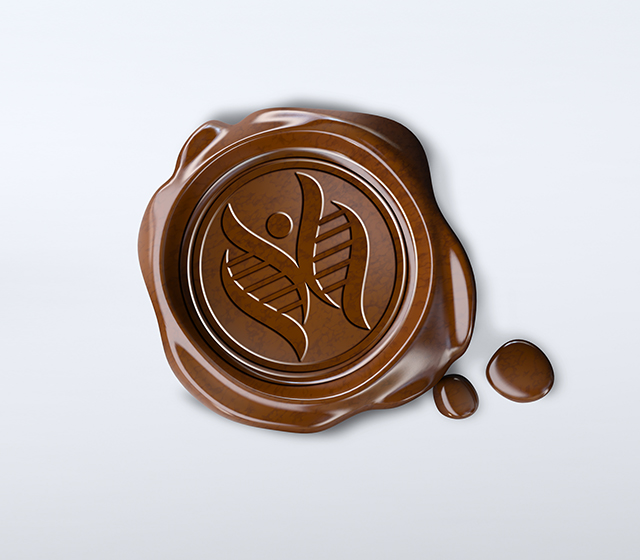
(责任编辑:佳学基因)